Today, image annotation is a cornerstone of AI development, powering systems that rely on accurate data to function effectively. As technology evolves, so do the methods for annotating visual data. This article explores the latest techniques in transforming image annotation, offering insights into how they improve workflows and deliver better results. Read on to discover what makes these innovations essential for advancing AI.
Understanding Modern Image Annotation
The rapid growth of computer vision applications in areas like autonomous driving and healthcare has highlighted the increasing importance of image annotation services. These services form the backbone of AI model training, enabling machines to interpret and process visual data effectively. In 2025, annotating images and videos will remain fundamental to AI development, especially as demand for real-time video annotation and 3D object detection continues to rise.
Traditional image annotation methods often relied on fully manual processes. While these techniques provided detailed annotations, they struggled to meet the demands of modern AI, where speed and scalability are essential. This gap paved the way for more advanced annotation approaches that integrate human expertise with automation.
Key advancements in image annotation include:
- Panoptic segmentation: Combining instance and semantic segmentation for a complete scene understanding.
- Semi-supervised learning: Leveraging smaller labeled datasets with larger unlabeled datasets to reduce manual effort.
- AI-assisted annotation tools: Accelerating annotation workflows with pre-labeling and prediction algorithms.
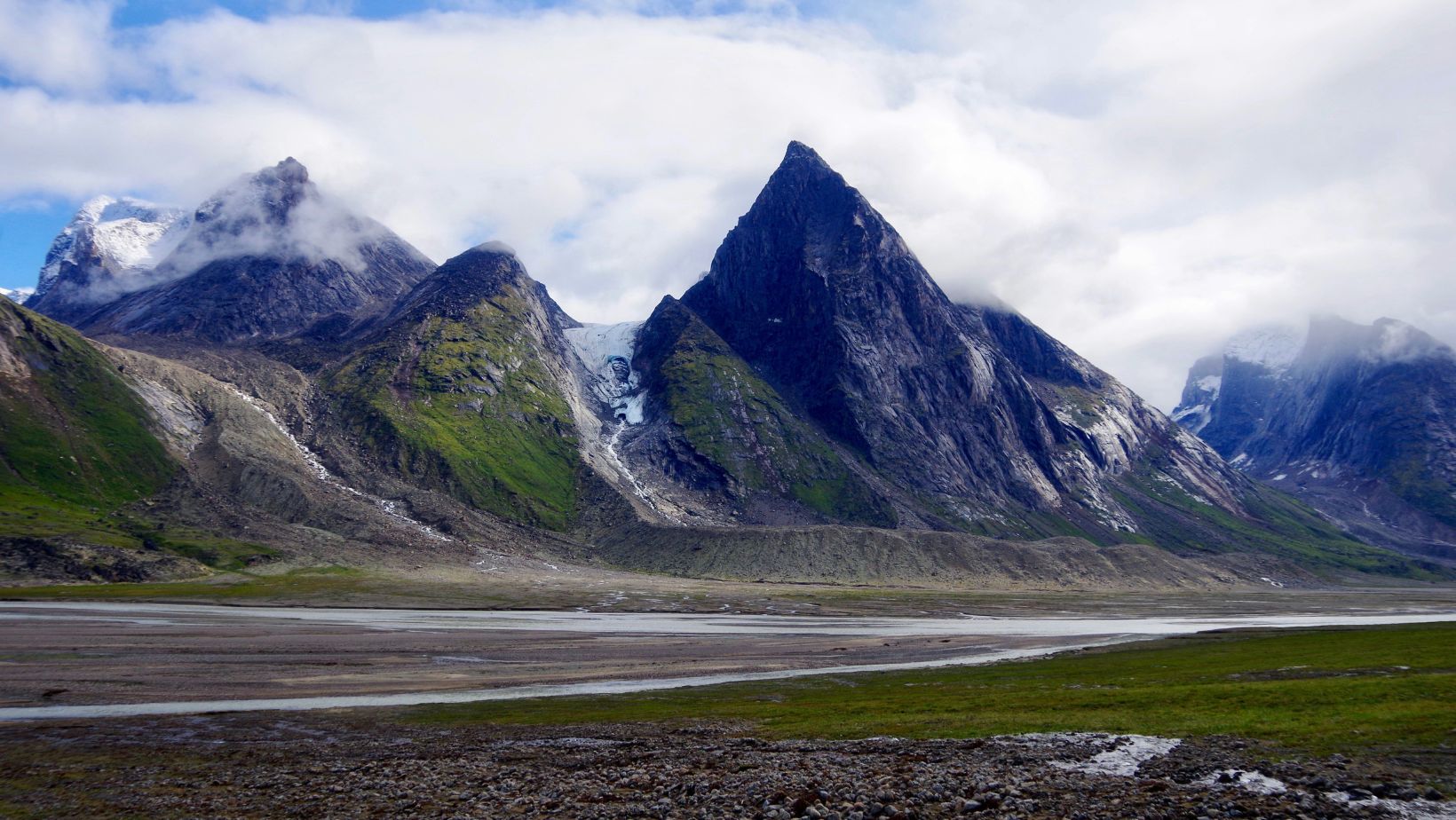
Each of these innovations improves efficiency and ensures higher-quality results. For instance, panoptic segmentation provides a holistic understanding of objects and their surroundings, which is vital for tasks like autonomous vehicle navigation. Similarly, semi-supervised learning reduces the dependency on fully annotated datasets while maintaining accuracy.
Understanding these modern approaches will allow your company to stay competitive in fields where annotated data drives decision-making. The shift from manual to hybrid solutions ensures that both accuracy and productivity are prioritized, creating opportunities for more robust AI models across industries.
Cutting-Edge Methods in Image Annotation
Advancements in technology have led to the development of innovative methods for image annotation. These approaches are designed to improve accuracy, save time, and handle large datasets more efficiently. As industries like healthcare and autonomous driving demand increasingly complex data annotations, these methods have become indispensable.
One of the most impactful advancements is panoptic segmentation, which combines semantic and instance segmentation. This method labels every pixel in an image, assigning categories to objects and distinguishing between individual instances. Panoptic segmentation is especially valuable in applications like urban planning and robotics, where understanding both the objects and their surroundings is necessary.
Another breakthrough is AI-assisted annotation tools. These tools use algorithms to pre-label data, significantly reducing the workload for annotators. While human oversight is still necessary to refine the results, these tools streamline workflows and improve productivity. They are especially effective in handling repetitive tasks, such as identifying objects in large datasets.
3D object detection also transforms image annotation. By creating three-dimensional representations of objects, this method enhances the precision of annotations in fields like virtual reality and autonomous vehicles. For instance, annotating depth and object dimensions is critical for training AI systems to navigate complex environments.
Other advanced techniques include:
- Active learning: Prioritizes annotating the most impactful data samples to improve model performance.
- Crowdsourced annotation platforms: Leverage a global workforce to manage large-scale projects.
- Semi-supervised learning: Uses a mix of labeled and unlabeled data to reduce manual effort.
These methods not only address scalability but also ensure higher quality annotations. For example, active learning focuses on refining datasets by targeting the most valuable data points, which leads to better model outcomes. Meanwhile, crowdsourcing allows businesses to tackle large annotation projects quickly and cost-effectively.
The integration of these cutting-edge methods is reshaping the way image annotation services operate. They enable organizations to meet the growing demand for annotated data while maintaining the accuracy needed for advanced AI applications. With continued innovation, these techniques will remain central to the evolution of data-driven technologies.
How to Use Innovative Techniques Effectively
To maximize the benefits of advanced image annotation methods, it’s essential to apply them strategically. Different projects require tailored approaches to ensure efficiency and accuracy. Here’s how organizations can effectively use these innovative techniques:
Steps to Optimize Image Annotation:
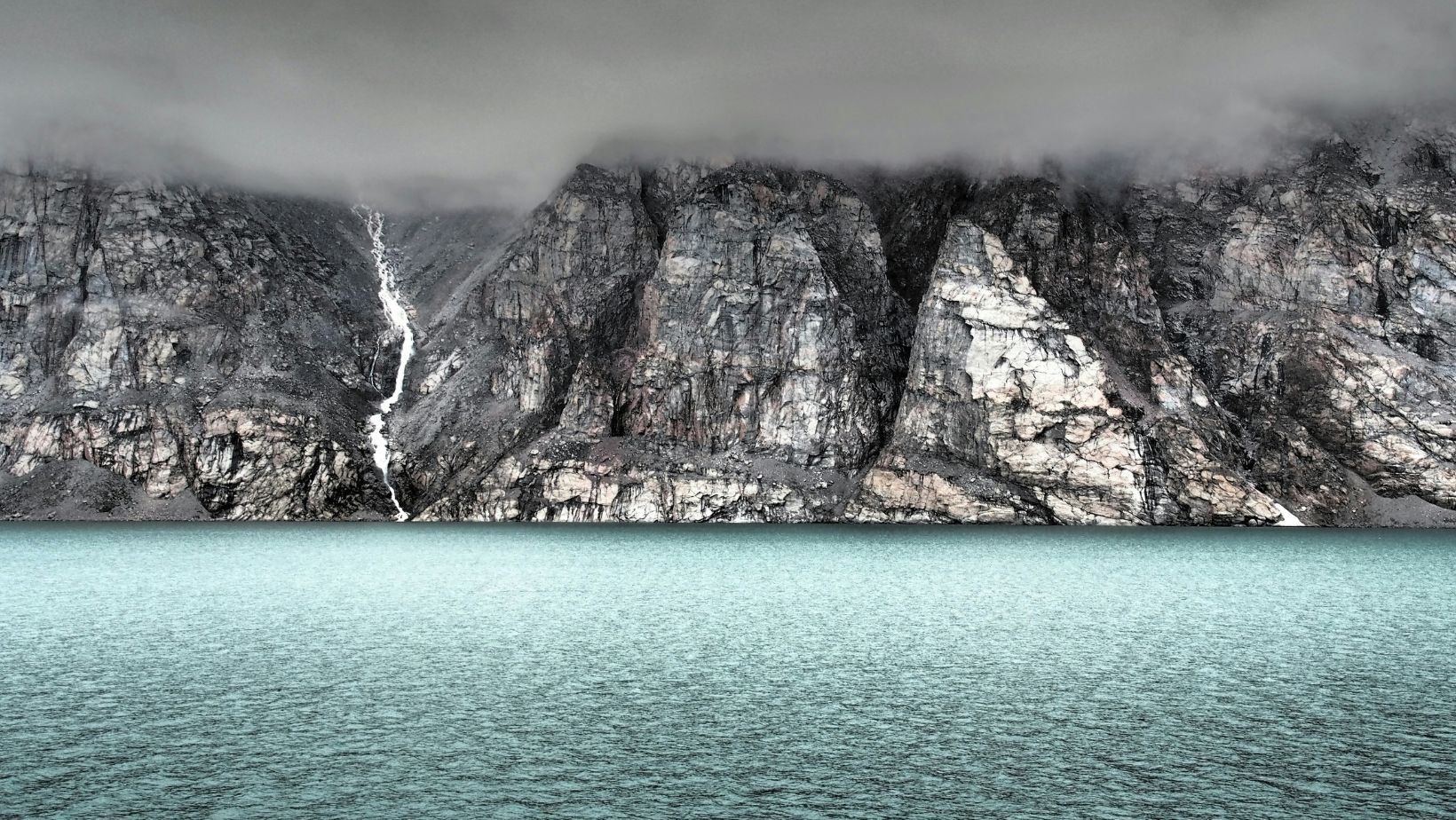
- Define project goals clearly: Identify what the annotated data will be used for. For instance, medical imaging requires precise segmentation, while autonomous vehicles need detailed 3D object detection.
- Choose the right tools: Select annotation tools that support advanced techniques like AI-assisted labeling or panoptic segmentation. These tools save time and ensure consistency.
- Combine automation with human oversight: Automated methods like active learning work best when human annotators review and refine outputs. This combination minimizes errors while improving speed.
- Prioritize data quality: Focus on annotating high-value data points first, especially in time-sensitive projects. Active learning helps determine which data samples are most impactful.
- Regularly validate results: Use quality assurance checks throughout the annotation process to ensure accuracy.
When applying these techniques, scalability is another critical factor. Projects that involve large datasets can benefit from crowdsourced platforms, which allow for faster completion without compromising quality. However, managing and training annotators on these platforms is essential to maintain standards.
Additionally, leveraging semi-supervised learning can reduce the need for fully labeled datasets. By combining small amounts of annotated data with larger unlabeled datasets, models can still achieve high accuracy with less manual effort.
Finally, continuously evaluate and update the annotation strategy based on project requirements. Emerging technologies may offer better alternatives, so staying informed about advancements ensures that workflows remain efficient.
What Is Next?
The future of image annotation lies in refining techniques and adapting to new challenges brought by evolving AI applications. As industries continue to demand more precise and scalable solutions, staying updated on the latest advancements and integrating them into workflows will be essential.
Innovative methods already show great potential, but their continuous improvement will define how effectively they meet the needs of complex AI systems. By focusing on strategic applications and embracing emerging technologies, you can remain at the forefront of this ever-changing field.